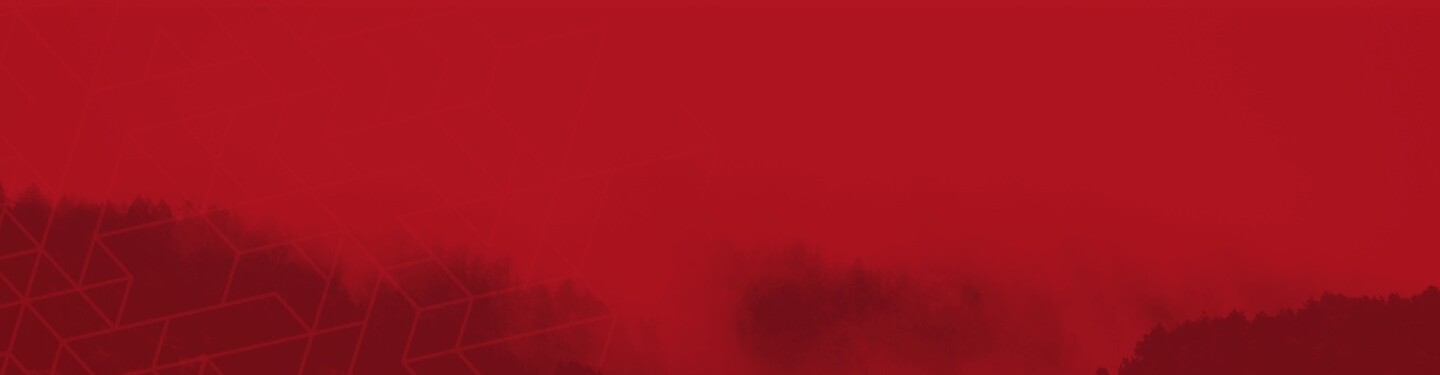
Understanding Alumni Communities Through Social Network Analysis
We tend to believe to be quite well informed about what is going on in our respective networks. We consider ourselves capable of assessing how vital some or all of our alumni communities are, whether the ties established are based on professional interests or personal friendships, and which individuals among the many alumni have become particularly well-connected.
However, these assumptions can be faulty and be based on our biased perceptions rather than valid data.
Building the Bosch Alumni Network, we questioned what we really knew about the various sub-networks we were aiming to connect. We wanted to stop guessing and base our strategic alumni work on more solid foundations.
Guiding questions included:
- How strongly connected are our alumni years after their program participation?
- Who are the influential individuals within the networks and how is this influence distributed?
- How "central" is the Robert Bosch Stiftung in those networks?
- What differences exist between various alumni networks?
- And most critically: What is the particular "glue" that holds our different alumni networks together and motivates them to stay engaged years after their program has ended?
To find the answers to these questions, we turned to Social Network Analysis.
How Social Network Analysis works: nodes & ties
Social Network Analysis is a data-driven, sociological approach that allows an analysis of the connections (so called "ties") between various actors in the network (so called "nodes").
To get to the right data, the process usually relies on a survey among a pre-defined group asking about different forms of connections, such as:
- How many connections are based on contacts?
- How does information travel?
- How high or low are levels of friendships within these groups?
Those completing the survey are simply asked to name those individuals they are connected with. Thus, it is possible to draw network maps using specific Social Network Analysis software.
For alumni networks of up to 60 individuals, it is possible to aim for a complete picture of the network. In this case, every single network member is asked to indicate his/her connection to all other network members.
If the network is larger, sub-categories are necessary, e.g. participants are given a list with names of their program year and asked to name ten additional people from the network they are regularly in touch with. This way, it is possible to get a fairly accurate picture of the network as a whole.
However, a very high response rate is usually necessary, and it is vital to ensure that everyone has given their consent to be part of the analysis. This requires extensive communication prior to the survey which in itself is already a great way to activate the network.
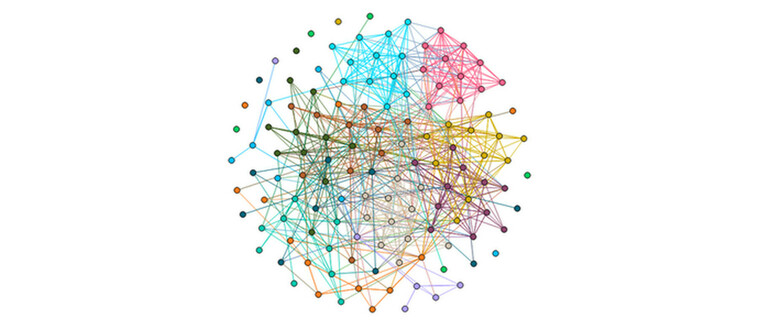
What the data can tell us
Beyond the visual overview that can already highlight some general patterns, Social Network Analysis allows us to dive much deeper. Key indicators helping to better understand the network include:
- Density: How dense is the network/how closely connected are the network members? Density is calculated by dividing the number of existing ties by the number of ties that are theoretically possible. In case of a 100% density, every individual within the network is connected to every other individual.
- In-Degree: Who are the people that were most often mentioned by others? These individuals are considered to be ‘Influencers’ simply because they are perceived by others as important. They can be a great resource if one seeks to influence the network because these influencers have great reach within the network.
- Out-Degree: Who are the people that indicated most connections to others? We consider these individuals to be ‘networkers’ because the more connections one actively seeks, the more reach - similar to influencers - this person has in the network.
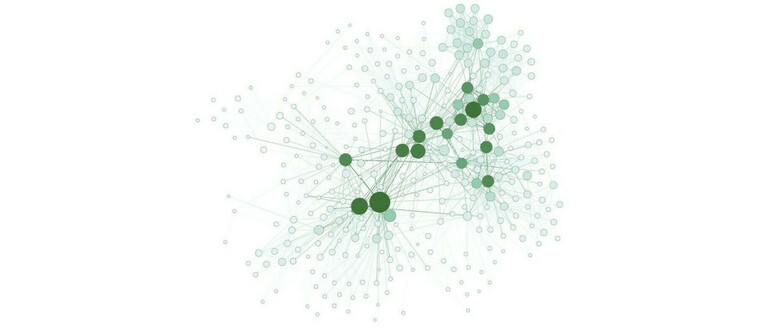
Working with these metrics brings more accuracy to the analysis and allows for a more strategic management of alumni communities. For instance, network density can serve as a benchmark to determine if activities to strengthen the network bear fruit. If after some months or years a network features greater density, this is a strong indicator of successful alumni activation. In/out-degree allows to zoom in on individuals who hold special roles in the network based on the connections they have.
Influencers and networkers both are key individuals to include when thinking about network interventions because those few can help reaching many others in the network.
Enriching data with context
To use of Social Network Analysis as a strategic tool, it helps to complement it with contextual information about the community. This data can be collected via the same survey and refer to, for example, the importance of the network for the alumni, what alumni want to contribute to the community, preferred event formats, or thematic issues high in demand. Knowing these contextual factors enables community managers to better address and involve alumni and to align future program offers, events and activities to the wishes of passive target groups.
Eventually, these measures will foster an inclusive, vital and cohesive alumni community that invites members to increase their interaction. Collected in multiple intervals, this data can be used to assess the long-time health and attractiveness of the community and monitor the success of strategic measures.
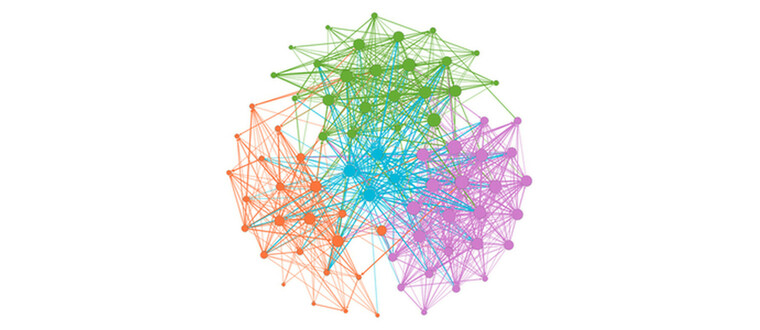
Key learnings for the Bosch Alumni Network
We have done four such analyses for the Bosch Alumni Network and created three key learnings:
- The way in which a program is set up ultimately imprints itself onto the structure of the alumni network. If you have a decentralized program which is managed from the outset and driven to a large extent by the program participants, your alumni network will reflect this. It will likely be self-sustaining and largely centered around contacts among alumni. In contrast, a program that is heavily guided and managed externally with little involvement of the program participants will likely also have an alumni network that revolves around the program's organizers.
- Our alumni network is most clearly structured along cohorts, thus, along the participant groups of programs. People are mostly in touch with people they have personally met and share a common experience with. This raises an important question: What does it take to encourage alumni to get in touch with other peers they haven’t met, yet? This question is particularly urgent for networks and communities that define themselves as a network of experts who support each other with their expertise and experience. The key is to ask alumni what they expect from their alumni network, how they want to engage and what is currently keeping them from engaging.
- Networks are held together by different "glues". A glue describes the underlying sentiments or incentives that compel alumni to engage with each other. For the Bosch Alumni Network, the potential career benefits were no convincing glue. Values and shared norms play a paramount role in our network instead. Being aware of the motivations and reasons that hold alumni together helps to design and offer suiting activities. In our case, we focus particularly on value-driven activities and on communicating those values.
Conclusion
Social Network Analysis provides accurate and empirically robust network maps and reveals patterns of relationships within the community in an intuitive, graphic way. It depicts the underlying structures of member interaction and indicates how dense and how intense networks are. It also serves as a tool to identify the most well-connected and the most trusted persons in a network that can serve as multipliers for future network activities.
Our experience shows that Social Network Analysis is particularly insightful when combined with contextual data about e.g. the experiences, expectations and motivations of the community members. Thus enriched, the analysis can serve to develop attractive offers and effective communication strategies that eventually lead to a vital and self-sustaining community.
This post is jointly written with Between|.|ness, a Berlin-based consultancy specialized in social network analysis and community building.
Do you want to find out more? Please feel free to contact:
Darius Polok
Managing Director
Darius.Polok@iac-berlin.org